After Meta, OpenAI, Microsoft, and Google – Alibaba Group is in the race for AI development to ease human life. Recently, Alibaba Group announced a new AI model, “EMO AI” – The Emote Portrait Alive. The exciting part of this model is that it can animate a single portrait photo and generate videos (talking or singing).
The recent strides in image generation, particularly Diffusion Models, have set new standards in realism. Majorly, AI models such as Sora, DALL-E 3, and others are developed on the Diffusion model. The Diffusion Models have significantly advanced, with their impact extending to video generation. While these models excel in creating high-quality images, their potential in crafting dynamic visual narratives has spurred interest in video generation. A particular focus has been on generating human-centric videos, such as talking head, which aims to authentically replicate facial expressions from provided audio clips. The EMO AI model is an innovative framework sidestepping 3D models, directly synthesizing audio-to-video for expressive and lifelike animations. In this blog, you will learn all about EMO AI by Alibaba.
Read on!
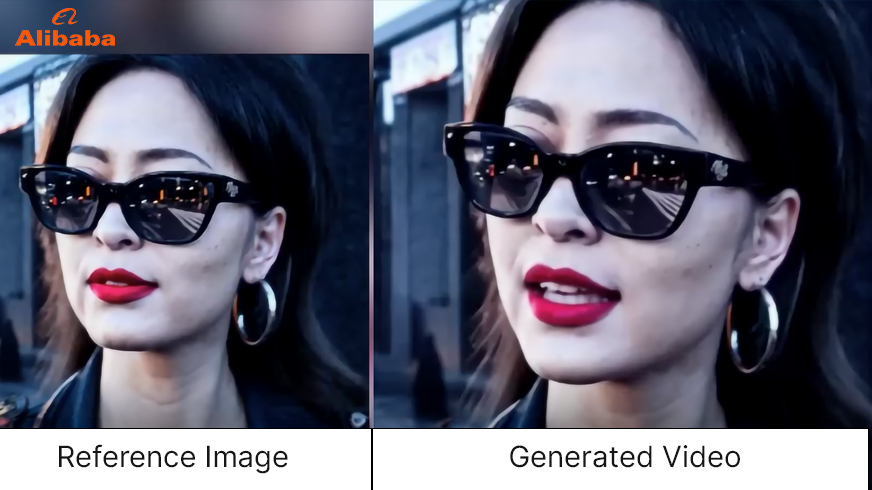
What is EMO AI by Alibaba?
Traditional techniques often fail to capture the full spectrum of human expressions and the uniqueness of individual facial styles,”. “To address these issues, we propose EMO, a novel framework that utilizes a direct audio-to-video synthesis approach, bypassing the need for intermediate 3D models or facial landmarks.
Lead Author Linrui Tian
EMO, short for Emote Portrait Alive, is an innovative system researchers at Alibaba Group developed. It brings together artificial intelligence and video production, resulting in remarkable capabilities. Here’s what EMO can do:
- Animating Portraits: EMO AI can take a single portrait photo and breathe life into it. It generates lifelike videos of the person depicted in the photo, making them appear as if they are talking or singing.
- Audio-to-Video Synthesis: Unlike traditional techniques that rely on intermediate 3D models or facial landmarks, EMO AI directly synthesizes video from audio cues. This approach ensures seamless frame transitions and consistent identity preservation, producing highly expressive and realistic animations.
- Expressive Facial Expressions: EMO AI captures the dynamic and nuanced relationship between audio cues and facial movements. It goes beyond static expressions, allowing for a wide spectrum of human emotions and individual facial styles.
- Versatility: EMO AI can generate convincing speaking and singing videos in various styles. Whether it’s a heartfelt conversation or a melodious song, EMO AI brings it to life.
EMO is a groundbreaking advancement that synchronizes lips with sounds in photos, creating fluid and expressive animations that captivate viewers. Imagine turning a still portrait into a lively, talking, or singing avatar—EMO makes it possible!
Also read: Exploring Diffusion Models in NLP Beyond GANs and VAEs
EMO AI Training
Alibaba introduced EMO, an expressive audio-driven portrait-video generation framework that directly synthesizes character head videos from images and audio clips. This eliminates the need for intermediate representations, ensuring high visual and emotional fidelity aligned with the audio input. EMO leverages Diffusion Models to generate character head videos to capture nuanced micro-expressions and facilitate natural head movements.
To train EMO, researchers curated a diverse audio-video dataset exceeding 250 hours of footage and 150 million images. This dataset covers various content types, including speeches, film and television clips, and singing performances in multiple languages. The richness of content ensures that EMO captures a wide range of human expressions and vocal styles, providing a robust foundation for its development.
The EMO AI Method
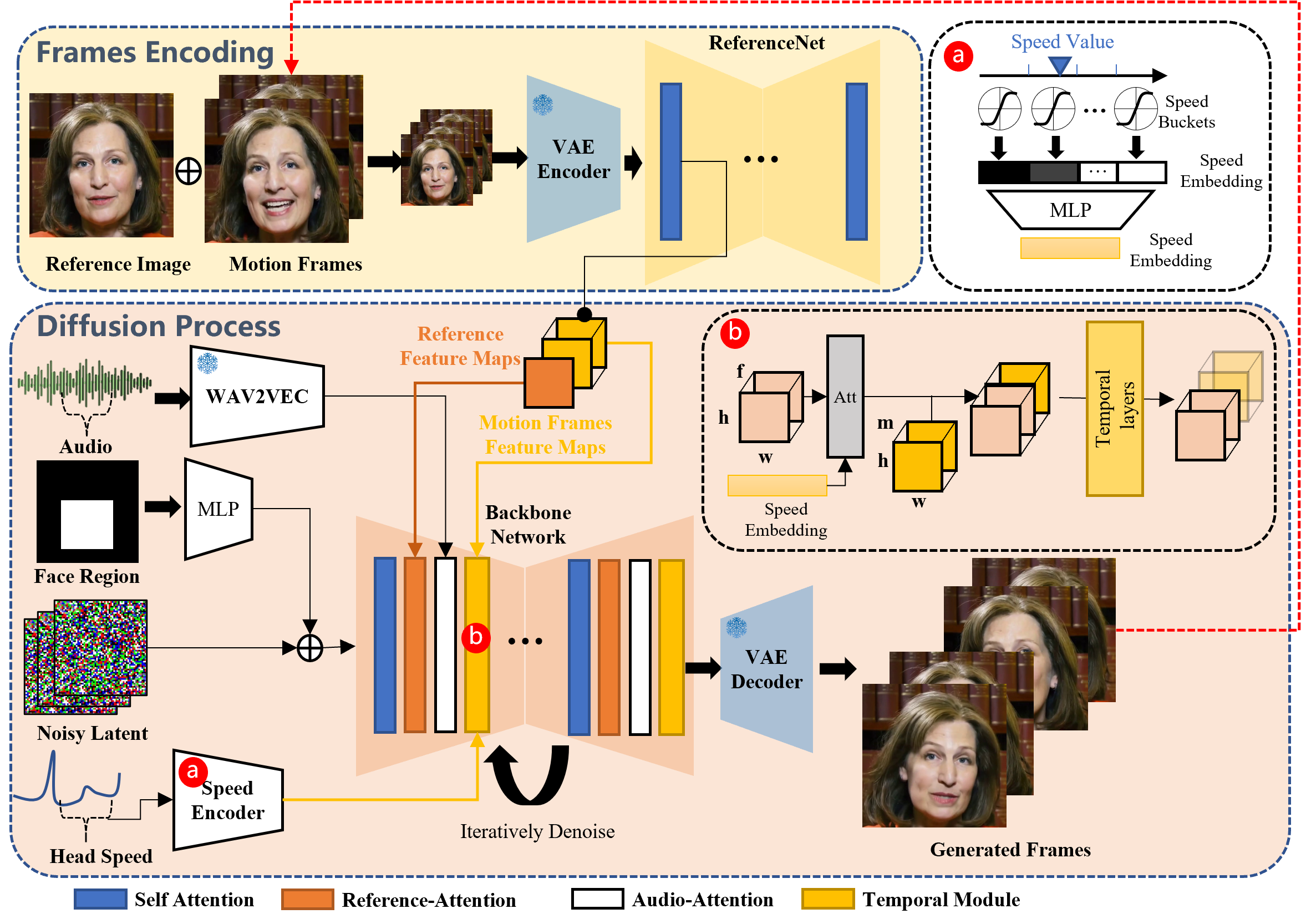
EMO’s framework comprises two main stages – frame encoding and the Diffusion process. In the Frames Encoding stage, ReferenceNet extracts features from the reference image and motion frames. The Diffusion Process involves a pretrained audio encoder, facial region mask integration, and denoising operations facilitated by the Backbone Network. Attention mechanisms, including Reference-Attention and Audio-Attention, preserve identity and modulate movements. Temporal Modules manipulate the temporal dimension, adjusting motion velocity for a seamless and expressive video generation process.
To maintain consistency with the input reference image, EMO enhances the approach of ReferenceNet by introducing a FrameEncoding module. This module ensures the character’s identity is preserved throughout the video generation process, contributing to the realism of the final output.
Integrating audio with Diffusion Models presents challenges due to the inherent ambiguity in mapping audio to facial expression. To address this, EMO incorporates stable control mechanisms – a speed controller and a face region controller – enhancing stability during video generation without compromising diversity. The stability is crucial to prevent facial distortions or jittering between frames.
Also read: Unraveling the Power of Diffusion Models in Modern AI
The Qualitative Comparisons
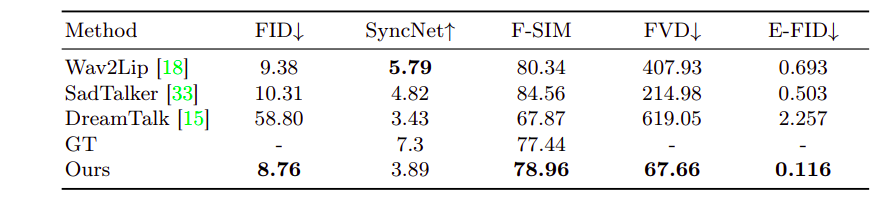
In the figure, you can find the visual comparison between the EMO method and previous approaches. When given a single reference image, Wav2Lip often produces videos with blurry mouth regions and static head poses, lacking eye movement. DreamTalk’s supplied style clips may distort original faces, limiting facial expressions and head movement dynamism. In contrast, the EMO method outperforms SadTalker and DreamTalk by generating a broader range of head movements and dynamic facial expressions. The EMO approach doesn’t utilize audio-driven character motion without relying on direct signals like blend shapes or 3DMM.
Results and Performance
EMO’s performance was evaluated on the HDTF dataset, surpassing current state-of-the-art methods such as DreamTalk, Wav2Lip, and SadTalker across multiple metrics. Quantitative assessments showcased EMO’s superiority, including FID, SyncNet, F-SIM, and FVD. User studies and qualitative evaluations further demonstrated EMO’s ability to generate natural and expressive talking and singing videos, marking it as the leading solution in the field.
Here is the Github link: EMO AI
Challenges with the Traditional Method
Traditional methods in talking head video generation often impose constraints on the output, limiting the richness of facial expressions. Techniques like using 3D models or extracting head movement sequences from base videos simplify the task but compromise naturalness. EMO aims to create an innovative framework that captures a broad spectrum of realistic facial expressions and facilitates natural head movements.
Check Out These Videos by EMO AI
Here are the recent videos by EMO AI:
Cross-Actor Performance
Character: Joaquin Rafael Phoenix – The Jocker – Jocker 2019
Vocal Source: The Dark Knight 2008
Character: AI girl generated by xxmix_9realisticSDXL
Vocal Source: Videos published by itsjuli4.
Talking With Different Characters
Character: Audrey Kathleen Hepburn-Ruston
Vocal Source: Interview Clip
Character: Mona Lisa
Vocal Source: Shakespeare’s Monologue II As You Like It: Rosalind “Yes, one; and in this manner.”
Rapid Rhythm
Character: Leonardo Wilhelm DiCaprio
Vocal Source: EMINEM – GODZILLA (FT. JUICE WRLD) COVER
Character: KUN KUN
Vocal Source: Eminem – Rap God
Different Language & Portrait Style
Character: AI Girl generated by ChilloutMix
Vocal Source: David Tao – Melody. Covered by NINGNING (mandarin)
Character: AI girl generated by WildCardX-XL-Fusion
Vocal Source: JENNIE – SOLO. Cover by Aiana (Korean)
Make Portrait Sing
Character: AI Mona Lisa generated by dreamshaper XL
Vocal Source: Miley Cyrus – Flowers. Covered by YUQI
Character: AI Lady from SORA
Vocal Source: Dua Lipa – Don’t Start Now
Limitations of the EMO Model
Here are the limitations:
- Time Consumption: The method employed has certain limitations, with one key drawback being its increased time requirement compared to alternative approaches not reliant on diffusion models.
- Unintended Body Part Generation: Another limitation lies in the absence of explicit control signals for directing the character’s motion. This absence may lead to the unintended generation of additional body parts, like hands, causing artifacts in the resulting video.
One potential solution to address the inadvertent body part generation involves implementing control signals dedicated to each body part.
Conclusion
EMO by Alibaba emerges as a groundbreaking solution in talking head video generation, introducing an innovative framework that directly synthesizes expressive character head videos from audio and reference images. Integrating Diffusion Models, stable control mechanisms, and identity-preserving modules ensures a highly realistic and expressive outcome. As the field progresses, Alibaba AI EMO is a testament to the transformative power of audio-driven portrait-video generation.
You can also read: Sora AI: New-Gen Text-to-Video Tool by OpenAI