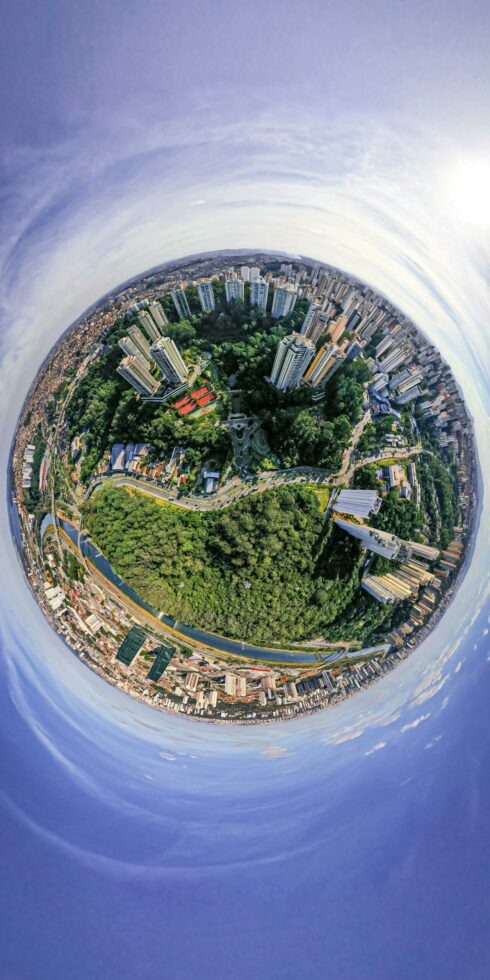
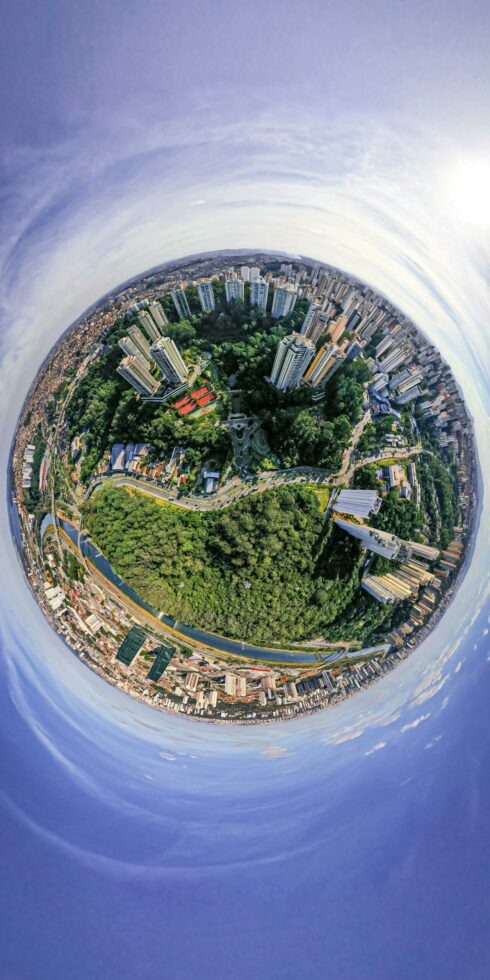
There are many reasons why duplicate entries might end up in a database, and it’s important that companies have a way to deal with those to ensure their customer data is as accurate as possible.
In Episode 5 of the SD Times Live! Microwebinar series of data verification, Tim Sidor, data quality analyst at data quality company Melissa, explained two different approaches that companies can take to accomplish the task of data matching, which is the process of identifying database records to link, update, consolidate, or remove found duplicates.
“We’re always asked ‘what’s the best matching strategy for us to use?’ and we’re always telling our clients there is no right or wrong answer,” Sidor explained during the livestream. “It really depends on your business case. You could be very loose with your rules or you can be very tight.”
RELATED CONTENT: Achieving the “Golden Record” for 360-degree Customer View
In a loose strategy, you are accepting the fact that you may be removing potential real matches. A company might want to apply a loose strategy if the end goal is to avoid contacting the same high-end client twice or to catch customers who have submitted their information twice and altered it slightly to avoid being flagged as someone who already responded to a rewards claim or sweepstakes.
Matching strategies for a loose strategy include using fuzzy algorithms or creating rule sets that use simultaneous conditions. Fuzzy algorithms can be defined as string comparison algorithms which determine if inexact data is approximately the same according to an accepted threshold. The comparisons can either be auditory likenesses or string similarities, and are a combination of publicly published or proprietary in nature. Rule sets with simultaneous conditions are essentially logically OR conditions, such as matching on name and phone OR name and email OR name and addresses.
“This will result in more records being flagged as duplicates and a smaller number of records output to the next step in your data flow,” Sidor explained. “You do this knowing you’re asking the underlying engine to do more work, to do more comparisons, so overall throughput on the process may be slower.”
The other alternative is to apply a tight strategy. This is best in situations where you don’t want false duplicates and don’t want to mistakenly update the master record with data that belongs to a different person. Using a tight strategy results in fewer matches, but those matches will be more accurate, Sidor explained.
“Anytime you need to be extremely conservative on how you remove records is when to use a tight matching strategy,” said Sidor. For example, this would be the strategy to use when dealing with individual investment account data or political campaign data.
In a tight strategy you would likely create a single condition compared to in the loose strategy where you can create simultaneous conditions.
“You wouldn’t want to group by address or match by address, you’d use something tighter like first name and last name and address all required,” said Sidor. “Changing that to first name and last name and address and phone number is even tighter. “
No matter which strategy is right for you, Sidor recommends first experimenting with small incremental changes before applying the strategy to the full database.
“Consider whether the process is a real-time dedupe process or a batch process,” said Sidor. “When running a batch process, once records are grouped, that’s it. There’s really no way of resolving them, as there might be groups of eight or 38 records in the group due to those advanced loose strategies. So you probably want to get that strategy down pat before applying that to production data or large sets of data.”
To learn more about this topic, you can watch episode 5 of the SD Times Live! microwebinar series on data verification with Melissa.