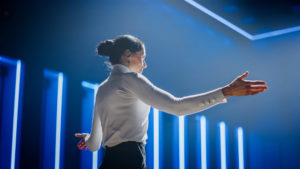
(Gorodenkoff/Shutterstock)
In the contemporary corporate landscape, the assessment of leadership impact on employee work-life balance transcends traditional measures, necessitating a multifaceted approach. Contemporary research underscores the profound influence of leadership on employee satisfaction, with a striking 77% of respondents attributing their job satisfaction to leadership behaviors. This statistic highlights the critical interplay between leadership styles and employee well-being.
In this context, the exploration of leadership impact is about quantifying and qualifying the nuances of this relationship which can be best done through Advanced Analytics. It is imperative to consider diverse leadership styles, each harboring unique potentials and challenges in fostering an environment conducive to optimal work-life balance.
The Importance of Data-Driven Metrics
The intricate balance between quantitative and qualitative metrics forms the bedrock of a sophisticated, informed leadership strategy. Organizations leveraging data-driven leadership reported a 23% increase in change success, underscoring the tangible impact of data-informed decision-making. This statistic exemplifies the dual role of data in leadership: not only does it guide strategic decision-making, but it also fosters a culture of transparency and trust, pivotal for employee buy-in and organizational resilience.
Employee Surveys and Self-Reports with AI and Advanced Analytics
In the realm of organizational dynamics, the role of AI and advanced analytics in employee surveys and self-reports is pivotal. These tools, including employee engagement surveys, are instrumental in capturing the multifaceted aspects of employee experiences. The integration of AI enables the processing of large datasets, providing nuanced insights into employees’ workplace connection, motivation, and alignment with company values. Advanced analytics further enhances the effectiveness of these surveys, ensuring high response rates and superior feedback quality through intelligent integration into regular communications.
The accuracy of survey results hinges on their reliability and validity. Reliability guarantees consistent outcomes over time, while validity ensures the survey measures precisely what it is designed to. Factors like question order and rating scales have significant impacts on data quality.
Key Performance Indicators (KPIs)
Key Performance Indicators (KPIs) remain crucial in providing insights into organizational performance, especially in the context of employee management and varying leadership styles. In 2023, the integration of AI and advanced analytics in data-driven HR analytics significantly enhanced the effectiveness of KPIs such as employee retention rates, absenteeism, and productivity metrics. A notable study revealed that 69% of large organizations now have a dedicated people analytics team, highlighting the growing reliance on these advanced tools for improving employee satisfaction, company culture, productivity, and revenue growth.
- Employee Retention Rates: AI-driven analytics significantly improve the assessment of leadership effectiveness in maintaining a stable workforce. Advanced algorithms analyze survey data, capturing nuanced employee sentiments toward leadership, competitiveness of compensation, and work-life balance. These analytics provide deeper insights, allowing organizations to tailor their leadership strategies or introduce targeted incentives to bolster retention rates.
- Absence Management: AI tools are instrumental in tracking and interpreting absenteeism data, offering comprehensive insights into employee well-being and engagement. By analyzing patterns in absenteeism, AI can identify potential workplace issues like stress, burnout, or dissatisfaction, which may vary under different leadership styles. This sophisticated analysis aids in proactive interventions to enhance the work environment.
- Productivity Metrics: The application of AI and advanced analytics in measuring productivity allows for a more accurate understanding of employee output and efficiency. These tools can discern subtle influences of various leadership styles on productivity, distinguishing between approaches that motivate and those that hinder employee performance.
The role of AI and advanced analytics is crucial in understanding how leadership styles impact these KPIs. For instance, AI can identify trends linking micromanagement with decreased employee retention or how a balanced leadership approach positively affects morale and retention rates. Similarly, advanced analytics can shed light on the effects of different leadership styles on productivity, distinguishing between hands-on and hands-off approaches.
Psychological and Health Metrics Enhanced with AI and Advanced Analytics
The integration of psychological and health metrics in the workplace, specifically those related to physiological stress markers and mental health diagnoses, is increasingly vital for employee well-being. AI and advanced analytics, backed by crucial statistical data, play a pivotal role in enhancing the effectiveness and precision of these metrics.
- Physiological Stress Markers and Mental Health Diagnoses
AI algorithms, equipped with statistical analysis, are employed to interpret complex patterns in physiological stress markers and mental health conditions. For instance, AI can statistically analyze trends indicating serious mental illness (SMI) or substance use disorders among employees. The economic impact of SMI in the United States, exceeding $300 billion annually, underscores the importance of this analysis. Through statistical correlations and pattern recognition, AI helps in identifying and addressing these issues more effectively in the workplace.
- Ethical Considerations in Health Surveillance
When implementing AI and advanced analytics in health surveillance, ethical considerations must be addressed, especially concerning data privacy and confidentiality. AI systems, utilizing statistical methods, can anonymize and securely manage sensitive health data, ensuring compliance with ethical standards. Additionally, statistical analysis helps in developing inclusive mental health solutions that consider various demographic factors, ensuring equitable access to mental health resources.
- Leadership Styles and Employee Health Metrics
Statistical analysis through AI and advanced analytics is instrumental in assessing the impact of different leadership styles on employee health metrics. By statistically analyzing data from surveys, performance metrics, and health indicators, AI can establish connections between specific leadership behaviors and their effects on employee mental health. For example, supportive leadership, characterized by empathy and assistance, has been statistically linked to positive mental health outcomes. In contrast, authoritative or neglectful leadership styles are often associated with higher levels of stress, absenteeism, and burnout, as evidenced by statistical trends in employee health data.
Utilizing statistical analysis, AI systems can discern the nuanced effects of leadership styles on workplace stress and overall job satisfaction. For instance, a leadership approach that emphasizes employee empowerment and open communication has been statistically correlated with lower stress levels and higher job satisfaction. Conversely, leadership styles that are overly authoritative or neglectful can statistically lead to an increase in stress-related symptoms and health issues among employees.
Case Studies and Comparative Analysis
The methodological approach to case studies and comparative analyses in organizational research has evolved with the adoption of the neo-configurational approach. This advanced method provides a more intricate understanding of organizational dynamics by emphasizing causal complexity. It moves away from traditional correlation-based methods, instead using set-theoretic relations to delve deeper into the causal relationships within organizations.
In terms of understanding employee experiences under different leadership styles, qualitative research methods are invaluable. They offer in-depth insights into employee perceptions and behaviors, crucial for assessing the impact of various leadership approaches on morale and job satisfaction.
Qualitative research, while providing detailed and contextual data, faces challenges in standardizing data analysis and ensuring generalizability. Its strength lies in its adaptability, making it an essential tool for exploring the complex dynamics of organizational behavior.
Final Reflections on Assessing Leadership Impact
To effectively assess leadership impact in today’s corporate environment, a multifaceted approach is essential. This approach balances quantitative data, like AI-enhanced KPIs and health metrics, with qualitative insights from advanced research methodologies. Such a comprehensive strategy is crucial for understanding the full spectrum of leadership’s influence on employee well-being. By embracing both data-driven insights and the nuanced perspectives offered by qualitative research, organizations can more adeptly navigate the complexities of leadership dynamics, thus creating environments that bolster employee satisfaction and drive overall organizational success.
About the author: Flo Lenoir is the Vice President of People Operations & Transformation at Infinit-O, where he guides agile teams to develop and leverage powerful technological tools to drive world-leading results in technology, financial, and healthcare services. His broad range of classical business education – with master’s degrees from ESC Rennes Business School in France, Ateneo De Manila in the Philippines, and Hanyang University in South Korea – provides a strong foundation to spearhead any team into the cutting-edge realm of processes optimization.
Related Items:
The Future of Workforce Analytics is Hidden in Your Communications
Workforce Analytics: How Big Data Is Shaping the Labor Pool
How AI Bolsters Job Recruiting with People Analytics