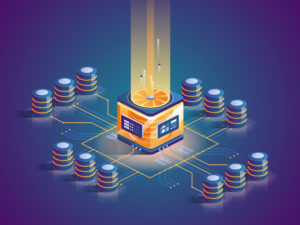
(Ico-Maker/Shutterstock)
Kubernetes, an open-source container orchestration system for automating software deployment, has had widespread adoption amongst organizations around the globe. However, accurately forecasting the resources needed by Kubernetes is often challenging, and can lead to operational risks, overprovisioning, resource wastage, and overspending.
For clusters containing 50 to 1,000 CPUs, organizations only use 13 percent of provisioned CPUs, and only around 20 percent of memory, on average, according to CAST AI, the leading Kubernetes automation platform for AWS, Azure, and GCP customers.
In the second annual Kubernetes Cost Benchmark Report released today, CAST AI analyzed thousands of real-world and active clusters running cloud-based applications. The report offers insights into cost optimization, cloud overspending, wasted resources, and other parameters.
The report is based on an analysis of 4,000 clusters running AWS, Azure, and GCP in 2023 before they were optimized by CAST AI’s automation platform.
One of the key findings of the report is that even for large clusters, CPU utilization remained low, which highlights that many companies running Kubernetes are still in the early stages of optimization. As more companies adopt Kubernetes, the cloud waste is likely to continue to grow.
“This year’s report makes it clear that companies running applications on Kubernetes are still in the early stages of their optimization journeys, and they’re grappling with the complexity of manually managing cloud-native infrastructure,” said Laurent Gil, co-founder and CPO, CAST AI. “The gap between provisioned and requested CPUs widened between 2022 and 2023 from 37 to 43 percent, so the problem is only going to worsen as more companies adopt Kubernetes.”
Interestingly, the CPU utilization trends are almost identical between AWS and Azure. They both have a utilization rate of 11 percent of provisioned CPUs. The cloud wastage was lowest on Google, at 17 percent.
For mega-clusters of 30,000 CPUs, the utilization becomes significantly higher at 44 percent. This is not surprising, as such large clusters tend to get a lot more attention from the DevOps teams managing them.
With the rising cloud service costs, reducing overspending has become more important than ever. Gartner forecasts worldwide end-user spending on public cloud services to grow by 20.4 percent in 2024.
The report shows that the biggest drivers of overspending include overprovisioning, where clusters are provided with more capacity than needed, and unwarranted headroom in pod requests, where memory requests are set higher than what Kubernetes applications require.
Another major cause of overspending is many organizations continue to be reluctant to use Spot instances. The number from the 2022 report shows that there have been no noticeable differences in Spot instances. This could be a quick and easy fix to improve CPU optimization.
CAST AI recommends using automation to provision the right size, type, and number of virtual machines (VMs). Many teams make the mistake of choosing instances they know and have used before, only to realize later that they are underutilizing the resources they have paid for.
There is a fine balance between overprovisioning and underprovisioning. If a team underprovisions resources they risk CPU throttling and out-of-memory issues which can lead to poor application performance. These issues can be resolved through automated workload rightsizing to match instance types and sizes to workload performance and capacity requirements.
Another recommendation by CAST AI is to autoscale nodes to fight CPU waste. While Kubernetes offers auto-calling features to increase utilization and reduce waste, the configuration and management of these tools are often challenging.
According to the report, using CAST AI to automatically replace suboptimal nodes with new ones can significantly boost optimization. Lastly, the report highlights the benefits of using Spot instances for cost savings.
The major concern about using Spot instances is the cloud provider can reclaim them on short notice causing unexpected downtime. This issue makes Spot instances appear risky. However, CAST AI believes they are stable and cost-effective. As long as you use automation to provision, manage, and decommission infrastructure, there should be no issues in using Spot instances.
Related Items
LTIMindtree Collaborates with CAST AI to Help Businesses Optimize Their Cloud Investments
The Three Approaches to AI Implementation