There have been numerous attempts to use AI to create 3D printable models based on a simple text input over the last year, with varying results. One of the more famous examples is from LumaAI, and you may recall this project from OpenAI earlier this year.
In this article we will take a look at another method of AI-powered text-to-3D, this time using a program called “Marigold”, which according to its website, “repurposes diffusion-Based image generators for monocular depth estimation”. Because generating AI art is one thing, but giving it actual depth, which is needed for 3D printing is an entirely different game altogether. But the creators of Marigold seem to have done just that.
What is Marigold?
Marigold was designed by researchers at ETH Zürich, and its function is to generate depth information from a 2D image. Originating from the Stable Diffusion framework, Marigold utilizes synthetic data for fine-tuning, enabling it to transfer knowledge to unfamiliar data efficiently. This approach has led Marigold to surpass the previously leading method, LeRes, in this field.
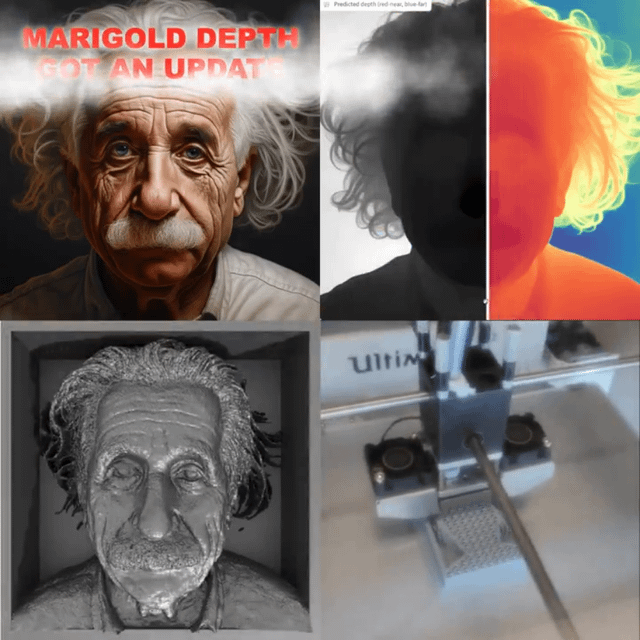
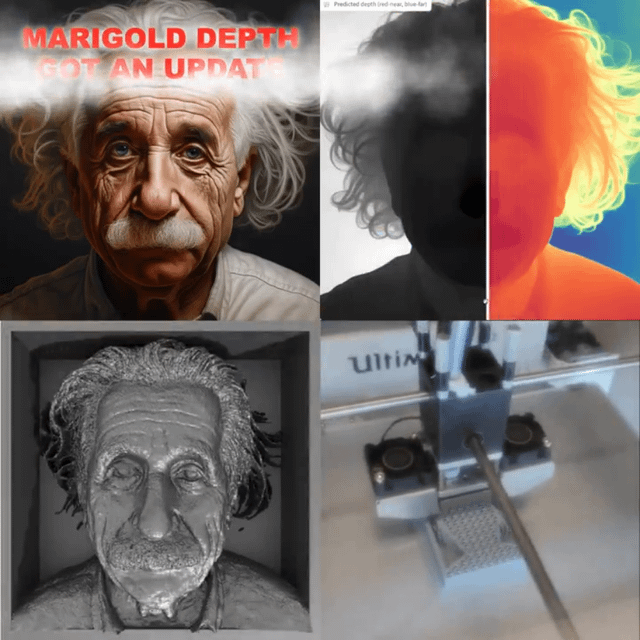
The model’s functionality hinges on fine-tuning the U-Net component of the Stable Diffusion setup. The process involves encoding both the image and depth into a latent space using the original Stable Diffusion VAE, followed by optimizing the diffusion objective relative to the depth latent code. A notable aspect is the modification of the U-Net’s first layer to accept concatenated latent codes, enhancing the depth estimation capability.
During inference, Marigold encodes an input image into a latent code, which is then concatenated with a depth latent. This combination is processed through the modified, fine-tuned U-Net. After several denoising iterations, the depth latent is decoded into an image, and its channels are averaged to derive the final depth estimation.
Comparative studies have shown Marigold’s superior performance in both indoor and outdoor settings against other state-of-the-art affine-invariant depth estimators. This is particularly notable as Marigold achieves these results without prior exposure to real depth samples. For a comprehensive understanding of Marigold’s methodologies and benchmarks, the detailed paper provides in-depth (no pun intended) information.
So because Marigold is an image-to-3D solution, it means that you can use AI generated images as the source. And that is exactly what one Redditor has done, as you can see below.
DallE-to-3D
Using the the DallE text-to-image AI found on Bing, Redditor “fredandlunchbox” entered a prompt to generate an image of a plastic picture frame with modern features, hanging on a wall.
DallE outputted the image that you can see below.
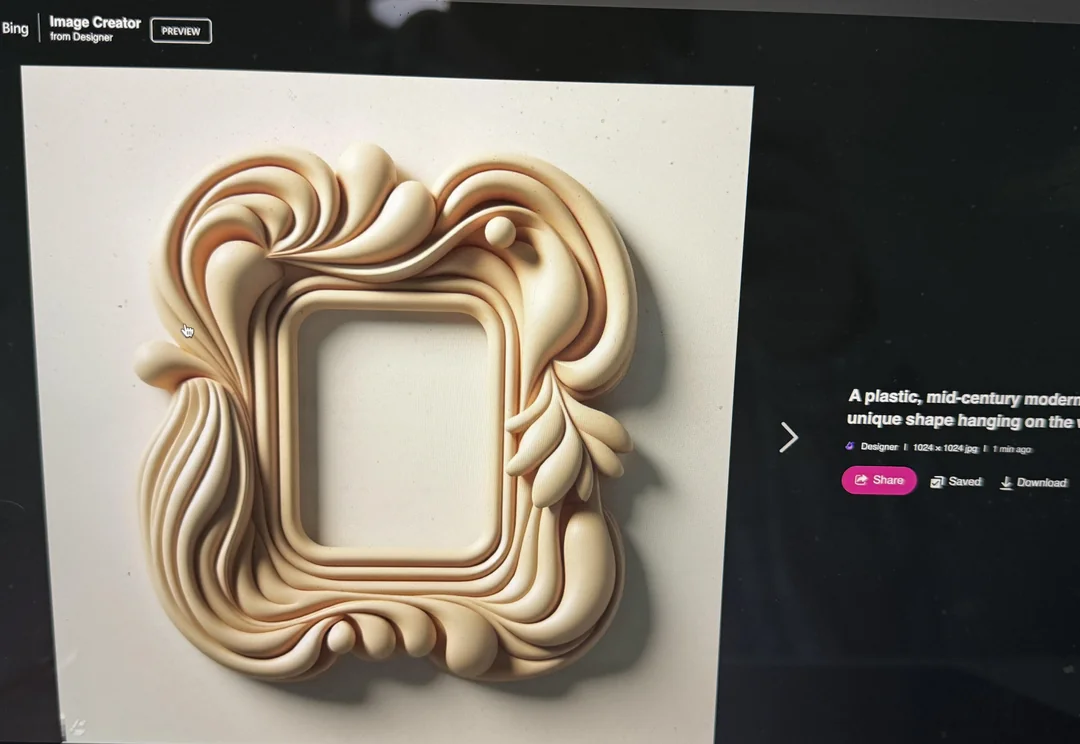
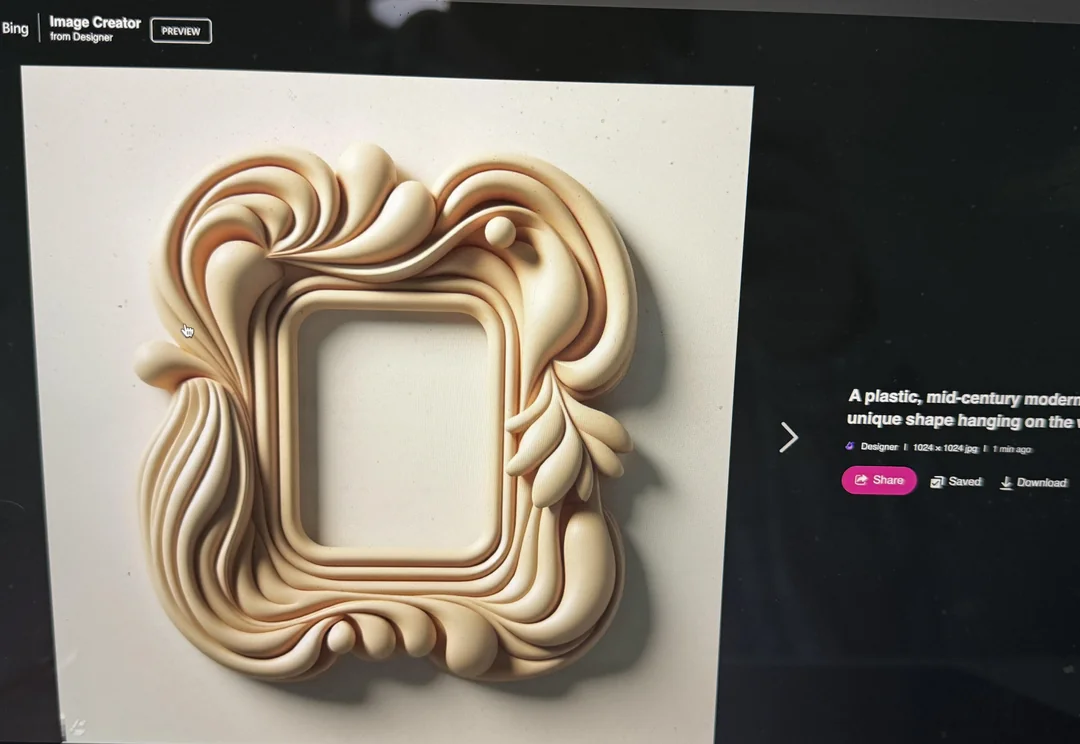
The Redditor used Marigold to create a depth map, before having the depth map rendered in Cinema4D, and then did some extra manual tidying up in Blender and Fusion360. When the model was complete, he exported it as an STL, and printed it. The whole process from DallE text-prompt to final printed object happened in under 24 hours, and you can see the final printed part in the image below.
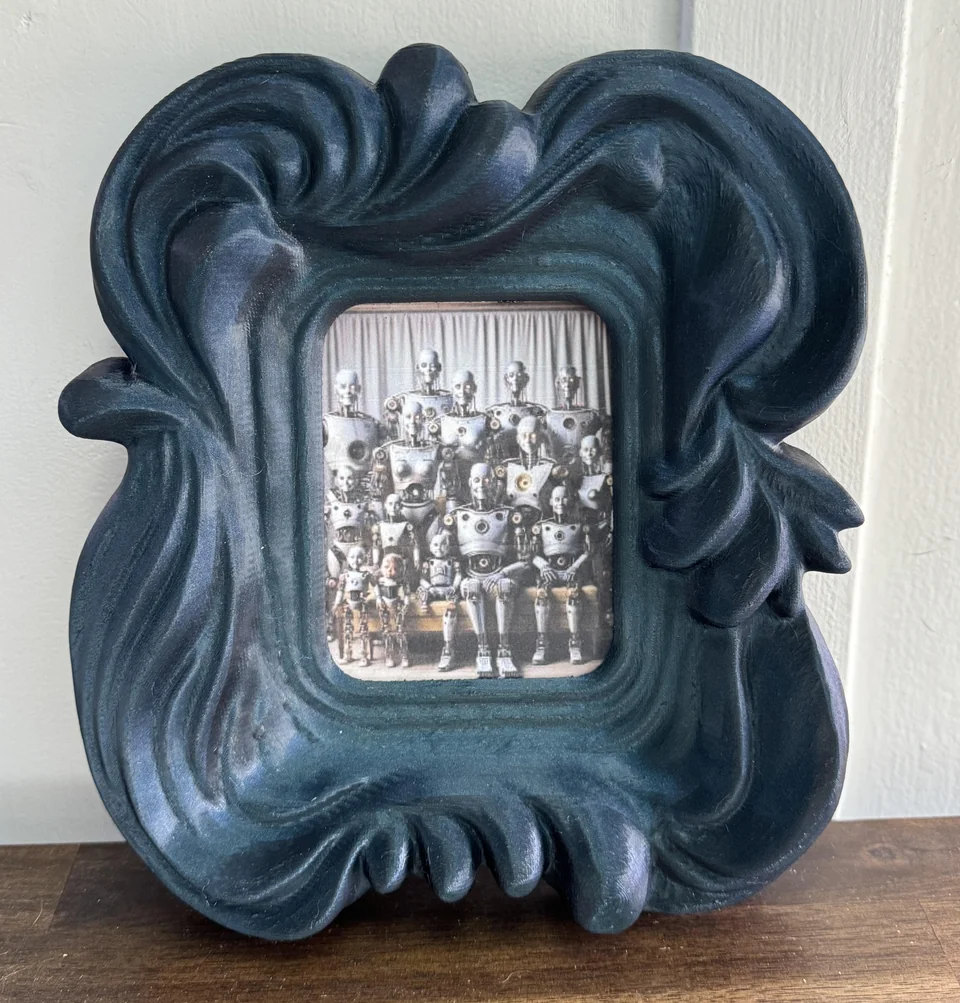
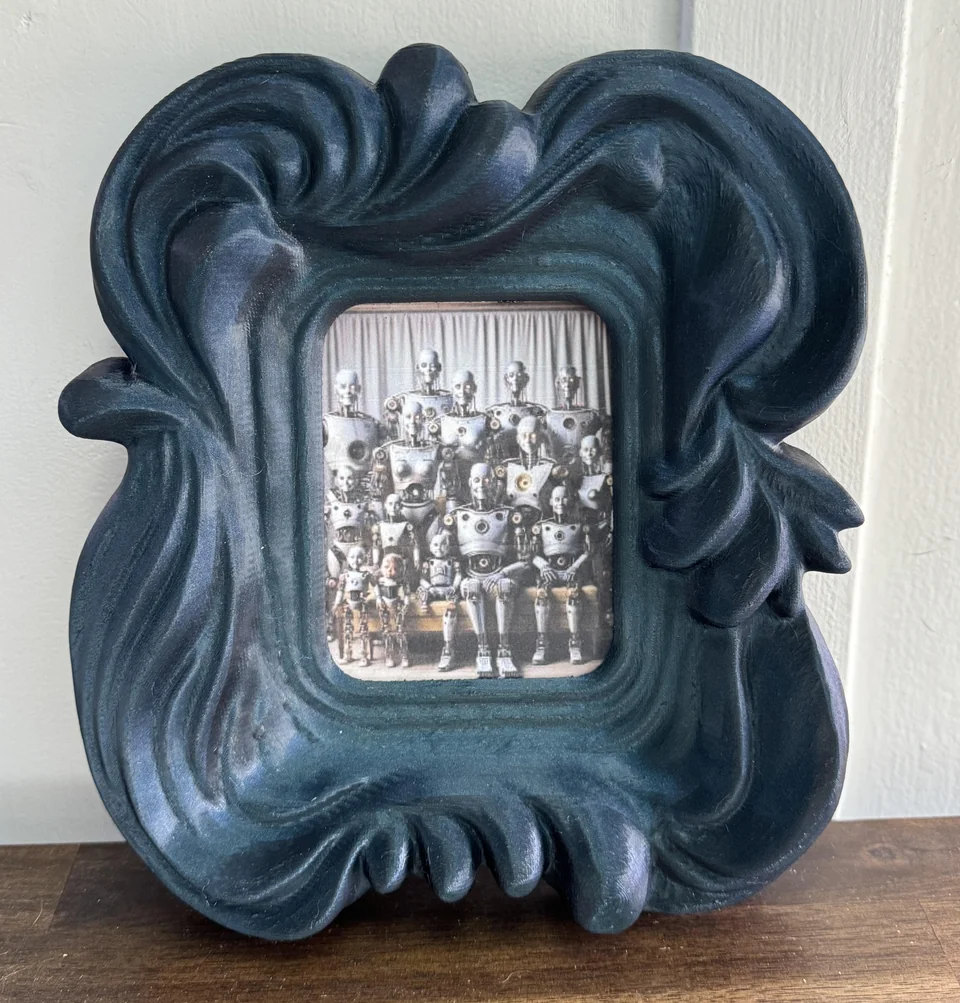
The Rdditor notes that the process is not automatic, but the creators of Marigold have recently announced an update that “allows the user to produce a watertight STL ready for printing.”
How watertight that is exactly, remains to be seen, but it sounds like something fun to experiment with over the holiday season.
If you would like to experiment with the depth estimation and 3D printing features of Marigold, you can head on over to the web-based GUI over at the program’s Hugging Face space over at this link.
Come and let us know your thoughts on our Facebook, X, and LinkedIn pages, and don’t forget to sign up for our weekly additive manufacturing newsletter to get all the latest stories delivered right to your inbox.